Matthew Kim
Student
LAHS ASI (Advanced Science Investigation) - Computer Science / Coding
Making The Future Safer: Artificial Intelligence and Subtle Biases
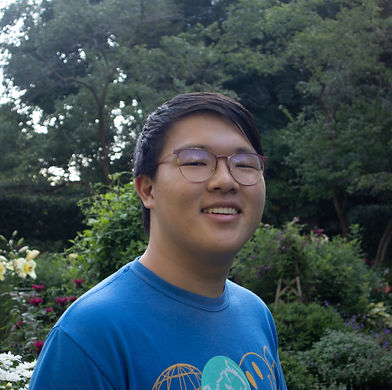
Technology
Research has found that NLGs are capable of generating toxic language, prompted or unprompted. However, there has been little such effort to moderate the AI generation of subtle hate in the form of microaggressions, subtle discriminatory remarks based upon one’s membership in an marginalized group. This project aims to identify the extent to which natural language models generate text-based microaggressions. This is done by first training an AI model capable of detecting microaggressions using a database of user-submitted microaggressions, as well as human-classified Reddit comments. The natural language model GPT-3 was then used to create ~10,000 AI generations, each roughly eighty characters long, using a corpus of sentence starters scraped from the internet, paired with toxicity scores from a commonly-used toxicity classifier. The aforementioned microaggression detection model was then used to classify each generation. Most classifications were identified as likely non-microaggressive, but a significant number of generations were identified as being likely microaggressive. However, empirical analysis finds that many (though not all) completions cited as potential microaggressions merely mentioned identity or one’s status in a marginalized group, meaning that more testing is necessary to draw conclusive results regarding AI’s ability to systematically generate microaggressions. In addition, there was little correlation between the toxicity of a prompt and how likely a generation was to be classified as microaggressive.
Meet the Speaker:
Matthew Kim is a LAHS senior who has been researching subtle biases in AI since last year. When he's not doing research, he loves writing poetry, solving Rubik’s Cubes and reviewing music.