Carter Capetz
Student
LAHS ASI (Advanced Science Investigation) - Chemistry
Polymers to Perovskites: The Possibilities of High School Research
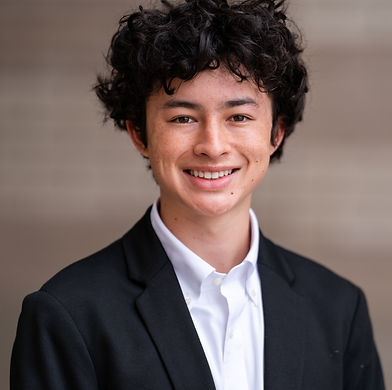
Science
Renewable Polymer: The purpose of this project is to explore polymer chemistry through the synthesis of a coblock polymer and gain insight into developing degradable polymeric materials to reduce plastic pollution. The procedure consisted of three main steps, including the ring-opening polymerization (ROP) of δ-decalactone, addition of L-lactide end block to poly(δ-decalactone), and precipitation and collection of the poly(L-lactide) poly(δ-decalactone) copolymer. Through experimentation, it was observed that the key to initiating the ROP of δ-decalactone was to prepare the correct solution. A 50/50 solution of 1M HCl and diethyl ether resulted in a cloudy polymer after the first step, and the copolymer did not solidify at the end of the second step. However, creating using catalytic HCl instead of 1M HCl solved the issue and led to an effective formation of the desired copolymer with acceptable yields. The resulting malleable polymeric material exhibited the desired degradation properties when exposed to heat or water for a longer period of time. Unfortunately, there was not enough time for NMR analysis, which could have shed more light on the copolymer's hydrolytic properties. Further research could explore similar monomers to δ-decalactone to see what properties of the materials would change. In conclusion, the synthesis of a renewable polymer from δ-decalactone and L‑lactide showed promising results in terms of degradability. This study provides insight into developing sustainable and environmentally friendly alternatives to conventional plastics.
Tensorized PINN: Physics-informed neural networks (PINNs) have seen increased utility due to their efficiency and accuracy when modeling complex physics systems, which are often governed by PDEs. To solve more complex PDEs or achieve better expressiveness, larger network architectures are required. Yet, the sensitivity of PINNs often results in ineffective compression. To resolve this problem, we propose a fully-tensorized PINN (ft-PINN) based on the Tensor-Train (TT) decomposition and tensorized training techniques. Additionally, we provide an exploration of PINN architectures to optimize compression ratios whilst maintaining or improving the model’s original expressiveness. To illustrate the benefits of our ft-PINN and advance past PDE solvers, we select and solve a non-linear PDE, the viscous Burgers' equation. Additionally, our ft-PINN accelerates both training and inferencing efficiency, maintains high accuracies, offers up to 98% overall parameter reduction, and significantly outperforms PINNs of similar size.
Meet the Speaker:
Carter started his research career through LAHS's Advanced Scientific Investigations (ASI) course, studying the synthesis and characterization of a renewable polymer from δ-decalactone and l‑lactide. Over the summer of 2023, he continued his research journey at UCSB, where he studied tensor compression techniques, enabling a physics-informed neural network (PINN) to run on resource-constrained edge devices. Now, he is pursuing research on improving Rapid Spray Plasma Processing (RSPP)-compatible perovskites with the use of ML to produce stable and efficient perovskite solar cells for commercial use.